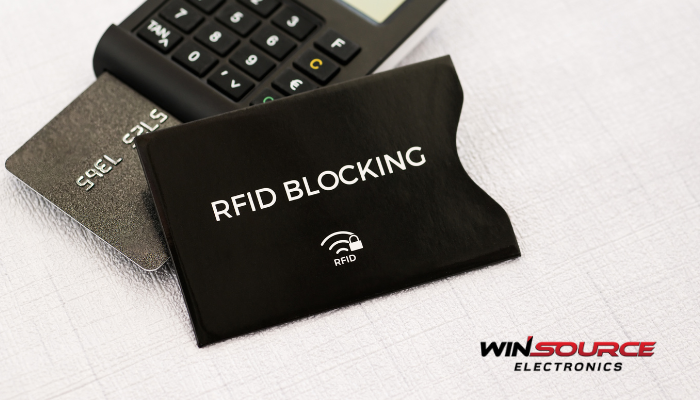
* Question
What are the main characteristics of pattern recognition of RFID data streams?
* Answer
Pattern recognition in RFID (Radio Frequency Identification) data streams is a crucial area of research and application, especially in the contexts of logistics, retail management, and security systems. RFID technology allows for the automatic identification and tracking of tags attached to objects. The data streams generated from RFID systems can be vast and complex, necessitating sophisticated pattern recognition techniques to derive useful information. Here are the main characteristics and challenges associated with pattern recognition in RFID data streams:
1. High Volume and Velocity: RFID systems can generate massive amounts of data due to the continuous reading of tags. Each tag read generates a new record, which includes the tag’s unique identifier, the reader’s identifier, and the timestamp. Handling this high volume and velocity of data in real-time is a significant challenge.
2. Noise and Redundancy: RFID data streams are inherently noisy and redundant. Multiple readings of the same tag can occur due to the proximity of tags to readers, and misreads or false reads are also common. Effective pattern recognition systems need to filter out noise and manage redundancy to avoid erroneous data interpretation.
3. Temporal and Spatial Data: RFID readings are not only about ‘what’ was read (the tag) but also ‘where’ and ‘when’. This spatial and temporal context is crucial for meaningful pattern recognition, such as tracking the movement of items through a supply chain or understanding shopping behaviors in retail.
4. Event Detection and Classification: Pattern recognition in RFID data streams often involves identifying significant events or classifying data patterns. For example, recognizing when items are misplaced, detecting unusual paths through a logistics network, or identifying bottlenecks in a production line.
5. Complex Data Integration: RFID systems often need to integrate data from multiple sources and sensors, including other RFID readers, GPS devices, or different types of sensors. Pattern recognition techniques must be able to handle and integrate diverse data types to provide a comprehensive view.
6. Real-Time Processing: Many applications require real-time or near-real-time pattern recognition to be effective. This includes applications like theft prevention, where immediate action is necessary, or inventory management, where real-time updates are crucial.
7. Privacy Concerns: Since RFID can be used to track movements of individuals (via tags on items they carry or wear), there are significant privacy concerns. Pattern recognition applications need to ensure compliance with privacy laws and regulations, often incorporating data anonymization techniques.
8. Adaptability and Learning: The effectiveness of RFID-based pattern recognition systems often depends on their ability to adapt to new patterns and changes in the environment. Machine learning techniques are frequently employed to improve the system’s adaptability and decision-making capabilities over time.
These characteristics define the requirements and constraints for developing effective pattern recognition systems in the context of RFID data. The solutions need to be robust, efficient, and capable of handling the complexity and scale of RFID-generated data, all while delivering actionable insights in a timely manner.
COMMENTS