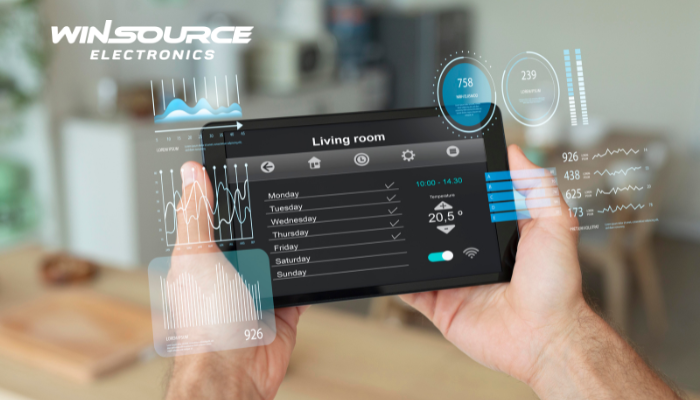
* Question
What are the main characteristics of pattern recognition of RFID data streams?
* Answer
Pattern recognition in RFID (Radio Frequency Identification) data streams is essential for extracting meaningful information from the vast and complex data generated by RFID systems. Here are the main characteristics and challenges associated with pattern recognition in RFID data streams:
1. High Volume and Velocity: RFID systems generate large volumes of data rapidly as each scan of a tag produces a record, which includes details such as the tag’s identifier, the time of reading, and the location of the reader. Managing and processing this high-volume, high-velocity data in real-time poses significant challenges.
2. Noise and Redundancy: RFID data streams are inherently noisy due to the nature of radio frequency communication and the environment in which RFID systems operate. Multiple readings of the same tag, misreads, and interference from other RF devices contribute to the redundancy and noise in the data, which necessitates robust noise filtering and data cleansing methods.
3. Temporal and Spatial Complexity: RFID data contains important temporal and spatial components as it tracks the movement of tags over time and across different locations. Pattern recognition algorithms need to effectively handle the dynamics of space and time to track movements, identify patterns, and detect anomalies.
4. Data Incompleteness and Inaccuracy: Not all tags may be read correctly or at every intended scan point, leading to incomplete or inaccurate data. Pattern recognition systems must be capable of dealing with missing data and drawing reliable conclusions from incomplete datasets.
5. Real-Time Processing Needs: Many applications of RFID technology, such as inventory management, supply chain monitoring, and security systems, require real-time or near-real-time processing of data to be effective. This demands efficient algorithms that can operate quickly and provide timely feedback.
6. Event Detection and Classification: Identifying meaningful events from raw RFID data streams, such as the entry or exit of goods in a warehouse, or irregular movements that might indicate theft or misplacement, is a core capability of pattern recognition systems in this context.
7. Integration with Other Data Sources: Effective pattern recognition often involves integrating RFID data with other data types from various sensors or systems, such as GPS data, environmental sensors, or databases. This integration can provide richer insights but also adds to the complexity of the data analysis.
8. Scalability: As the deployment of RFID systems expands, the pattern recognition solutions must scale accordingly, both in terms of handling larger datasets and maintaining performance under increased load.
9. Privacy and Security: RFID systems can potentially track individual movements or reveal sensitive information. Pattern recognition applications must address privacy and security concerns, ensuring that data handling complies with legal standards and ethical norms.
These characteristics highlight the need for sophisticated pattern recognition techniques and robust system designs to effectively leverage RFID data in practical applications.
COMMENTS